How Manolin’s Advanced Research Approach Reveals Real Impact on Salmon Farm Performance

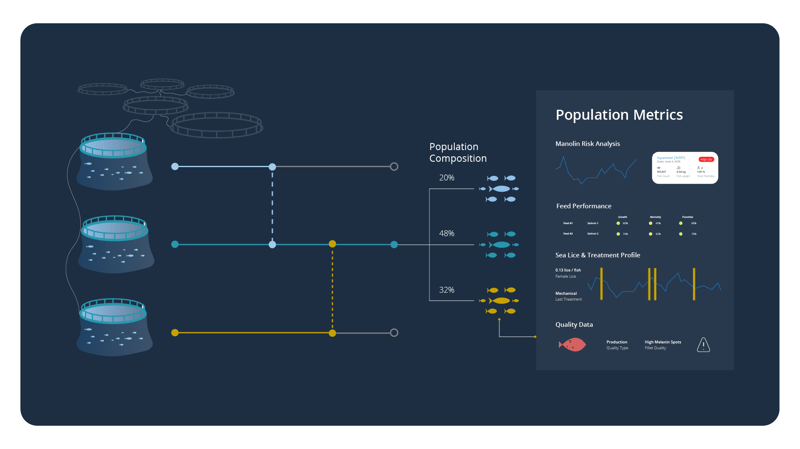
Over the last few years, we’ve seen a major shift in how farms think about data. It's no longer enough to simply collect it - the value comes from using it.
That’s why we’re excited to share some of the work behind our newest study with Veramaris, exploring the commercial impacts of different EPA and DHA feed levels on salmon performance worldwide.
You can download the full white paper for detailed results. But here, I want to dive into something just as important: the approach.
Because while the findings are valuable, it’s the methodology — and what it represents for aquaculture’s future — that could be even more transformative. Let’s take a closer look at how new tools like traced populations and advanced causal modeling are helping the industry move from averages to actionable, farm-specific insights.
Why We Took a Different Approach
Fish nutrition has become incredibly sophisticated over the last decade. Farmers have more feed options than ever — functional feeds, alternate lipid sources, tailored pigment levels — all designed to optimize fish health and efficiency.
At the same time, farms are collecting more data: mortality rates, treatments, feed rates, environmental metrics. Yet despite all this information, decision-making remains difficult.
The reality is:
-
Farms don’t operate in controlled environments.
-
No two production cycles are identical.
-
And data, if not analyzed properly, often raises more questions than it answers.
When Veramaris approached us to explore the real-world impact of EPA and DHA feed levels, we knew traditional methods wouldn't be enough. Academic studies offer controlled insights — but commercial farms deal with a messy, unpredictable reality.
So the challenge became: How can we extract clean, causal insights from complex, real-world operations? That meant developing a study that was both scientifically rigorous and grounded in the operational reality of salmon farming.
The Study Results And Why They Matter
Before getting into the methods, here’s a snapshot of what we found:
-
Populations fed above-average EPA/DHA levels (more than 9% of total fatty acids) showed:
-
8% lower mortality
-
13% better eFCR (economic feed conversion ratio)
-
Higher consistency and predictability across key performance metrics
-
These results weren’t drawn from lab trials or isolated farms. They came from a dataset covering over 430 million smolts and 55,000 fish generations across real-world, commercial environments.
That’s what makes this project significant - not just the outcome, but how we achieved it.
The Problem with Traditional Farm Data
If you’ve worked with production data, you know: it’s messy.
Fish move between pens. Conditions shift day to day. Mortality events, lice treatments, vaccinations — they don't happen uniformly.
Analyzing site averages or static KPIs often obscures what's really happening. It becomes almost impossible to separate the effect of a feed type from all the noise.
That’s why we introduced a new concept into the analysis: Traced Populations.
Instead of analyzing farms at the site level, we traced specific groups of fish — from smolt stocking to harvest — through all the environmental exposures, treatments, and management events they experienced.
Each traced population represented a coherent, biologically meaningful group:
-
Same stocking batch
-
Same exposure history
-
Same treatment protocols
This allowed us to:
-
Reduce variability caused by unrelated factors
-
Match populations across farms and regions more meaningfully
-
Isolate the true impact of feed levels on outcomes
It’s a critical shift: Instead of asking, "How did this farm perform?"
We can now ask, "How did this specific group of fish — under these specific conditions — perform?"
Borrowing from Medicine: How IPWRA Strengthens Causal Inference
Even with traced populations, one challenge remained: Farms don’t randomly assign feed types. Management strategies, risk profiles, customer requirements — all influence decisions.
If we simply compared high-EPA/DHA farms to low-EPA/DHA farms, we risk confounding the results. Maybe the farms using higher EPA/DHA were already better managed in other ways.
To overcome this, we borrowed a tool from modern healthcare analytics: Inverse Probability Weighted Regression Adjustment (IPWRA).
IPWRA is used to draw causal inferences from observational data — when randomized control trials aren’t feasible.
A great example comes from COVID-19 vaccine research during the pandemic. Studies couldn't ethically randomize pregnant individuals into vaccine and non-vaccine groups. Instead, researchers modeled the probability of vaccination based on each patient’s characteristics, then adjusted outcomes to estimate true vaccine effects.
We applied the same idea to aquaculture:
-
Modeled the probability that a traced population received a certain EPA/DHA feed level based on location, company, season, and other variables
-
Weighted and adjusted outcomes based on those probabilities
-
Estimated the true impact of different feed strategies — as if they had been randomly assigned
In short - We corrected for bias. And we did it using real farm data, not experimental trials. This opens massive new opportunities because farms don’t have to wait for perfect studies anymore. They can learn from the data they already generate.
Why This Matters Beyond EPA and DHA
The implications of this work go far beyond fatty acid levels.
By combining traced populations with causal modeling techniques like IPWRA, farms and suppliers can now:
-
Compare different lice treatment strategies
-
Evaluate the impact of environmental variables (like DO, temperature)
-
Benchmark health outcomes across production sites
-
Identify best practices based on their actual operational data, not generalized assumptions
It’s a major evolution from broad benchmarking toward personalized aquaculture insights.
Farms operate in unique biological and operational environments. Data science should reflect that complexity - not flatten it.
Looking Forward: Smarter Aquaculture Starts Here
This study with Veramaris proves that advanced statistical methods - once reserved for healthcare and human population studies - are ready for aquaculture.
It’s no longer just about collecting data. It’s about harvesting insight from it.
The ability to trace populations, model probabilities, and isolate true drivers of performance will become foundational for farms that want to:
-
Improve fish welfare
-
Increase efficiency
-
Reduce risk
-
Outperform under tightening regulatory and economic pressure
And we’re only scratching the surface.
With these tools, we can now start layering in genetic factors, environmental stressors, disease dynamics, and even operational decisions - building an intelligence system tailored for each farm’s unique context.
It’s an exciting time for aquaculture.
The future belongs to farms that don’t just collect data - but put it to work.