Model-Centric vs. Data-Centric AI: Transforming Fish Farms With Smarter, Cleaner Data
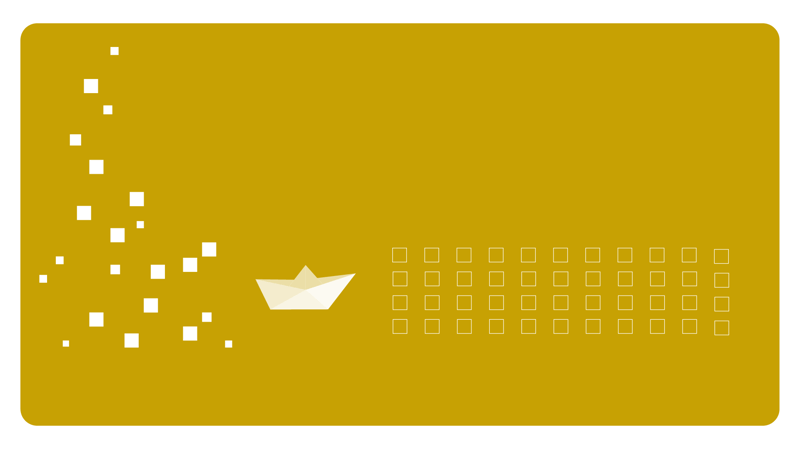
When it comes to designing effective AI solutions, two fundamental approaches exist: model-centric AI and data-centric AI. We break down the difference between these two approaches and explain why data-centric AI holds greater potential for aquaculture, particularly when applied to Manolin's AI data modeling.
What is Model-Centric AI?
Model-centric AI focuses on improving the performance of AI systems by optimizing algorithms and models. In this approach, the dataset is fixed, and all the effort is spent tweaking and fine-tuning the model itself to achieve better results. The primary challenge here is building a model sophisticated enough to handle a wide variety of data inputs and generate accurate predictions.
Example of Model-Centric AI in Aquaculture:
In a model-centric approach, a fish farm might develop an AI model to predict sea lice outbreaks. The focus would be on designing a robust machine learning model that processes environmental variables (like water temperature, salinity, or current speed) to make predictions about when and where sea lice are most likely to appear. In this scenario, improving the AI model is key, while the dataset used to train the model remains largely unchanged.
Challenges: In this model-centric system, the AI might struggle with accuracy if there are inconsistencies or biases in the data. The model could be highly optimized but still generate poor predictions if the underlying data is not representative of real-world conditions.
The Shift to Data-Centric AI
Data-centric AI flips this approach by focusing on improving the quality of data rather than the complexity of the model. Instead of constantly tuning the model, the idea is to ensure that the dataset is highly accurate, diverse, and well-labeled, allowing a simpler model to perform effectively. This approach is particularly valuable in industries like aquaculture, where the data itself is often variable and complex.
By enhancing data quality, data-centric AI allows models to work with cleaner, more representative datasets, leading to improved results without the need for overly complex algorithms.
Example of Data-Centric AI in Aquaculture:
A data-centric approach in aquaculture might involve improving the accuracy and consistency of data collected from various sensors and tracking systems on a farm. For example, farms could gather more consistent sea lice counts, precise water quality data, and environmental conditions across multiple locations. Rather than continuously upgrading the AI model itself, the focus shifts to ensuring that the dataset is comprehensive and clean.
This leads to more reliable predictions, as the improved data quality ensures that the AI model can learn from accurate and representative information.
Aspect | Model-Centric AI | Data-Centric AI |
Focus | Improving and tuning the AI model itself | Enhancing data quality, consistency, and relevance |
Data Handling | Data is fixed or minimally adjusted | Data is cleaned, enriched, and continually improved |
Performance | Gains from refining model architecture | Gains from improving data accuracy and relevance |
Cost & Efficiency | Often resource-intensive (model tuning, higher compute) | More cost-effective through improved data pipelines |
Real-World Usability | Limited by noisy and incomplete data | Better suited for complex, variable data environments |
Scalability | New models needed for each task or domain | Generalizable; adaptable across multiple models and domains |
Transparency | Less transparent, often "black-box" model complexity |
Manolin’s Data-Centric Approach to AI Modeling
At Manolin, we embrace a data-centric AI approach to build more reliable industry models. Let’s use our disease models as an example. Disease outbreaks are complex, multi-variable problems, with no single factor acting as a clear cause. Manolin’s software collects and integrates data from multiple sources—fish health records, environmental factors, and farm-specific trends—ensuring that our models have access to high-quality, cleaned, and well-organized structured data.
By focusing on improving the data, our platform allows for more precise insights and better predictions about disease outbreaks. Here's how we apply this data-centric approach:
Integrated Data Sources: Our disease models pull data across an array of farm data sources, ensuring that no critical information is missing.
Data Quality Management: We use self-healing data modules to continuously clean and optimize our datasets. This ensures that the models operate on high-quality, trustworthy data.
Feature Extraction and Relevance: Our models automatically identify the most important variables (e.g., temperature fluctuations, feeding patterns) to ensure that predictions are based on the most relevant factors, improving the model’s predictive capabilities.
Because we prioritize data quality, Manolin’s models are able to deliver more accurate predictions, helping farms prevent disease outbreaks and improve fish health management.
Why Data-Centric AI is the Future of Aquaculture
The success of AI systems, especially in industries like aquaculture, relies not just on building complex models but on feeding those models with the right data. Data-centric AI is particularly suited to aquaculture because the industry operates in highly variable environments, where conditions can change rapidly and unpredictably. The ability to extract and clean high-quality data is far more valuable than over-engineering a model to fit incomplete or messy data.
By focusing on data quality and integration, data-centric AI can unlock greater accuracy in predictions, enabling farms to make smarter, more informed decisions about fish health, disease prevention, and operational efficiency.
Why Aquaculture Needs Data-Centric AI
While model-centric AI has traditionally been the focus, it’s clear that the future of aquaculture predictions and fish health management lies in data-centric AI. By improving the quality of data feeding into models, farms and aquaculture companies can achieve more accurate, actionable insights with fewer resources.
At Manolin, our disease models prioritize data quality, enabling better predictions and smarter decisions. In an industry where environmental conditions and biological factors are constantly shifting, data-centric AI offers the most reliable path forward for achieving sustainable growth and optimizing fish health.